Our paper “CORE: Towards Scalable and Efficient Causal Discovery with Reinforcement Learning” got accepted at AAMAS 24.
I am excited to share that our paper “CORE: Towards Scalable and Efficient Causal Discovery with Reinforcement Learning” got accepted as a full paper at AAMAS24 . Thanks to Nicolò Botteghi, Erman Acar and Aske Plaat for making this stimulating collaboration a success.
In this work, we develop a deep reinforcement learning-based approach for causal discovery and intervention planning. Our approach learns to sequentially reconstruct causal graphs from data while learning to actively perform informative interventions.
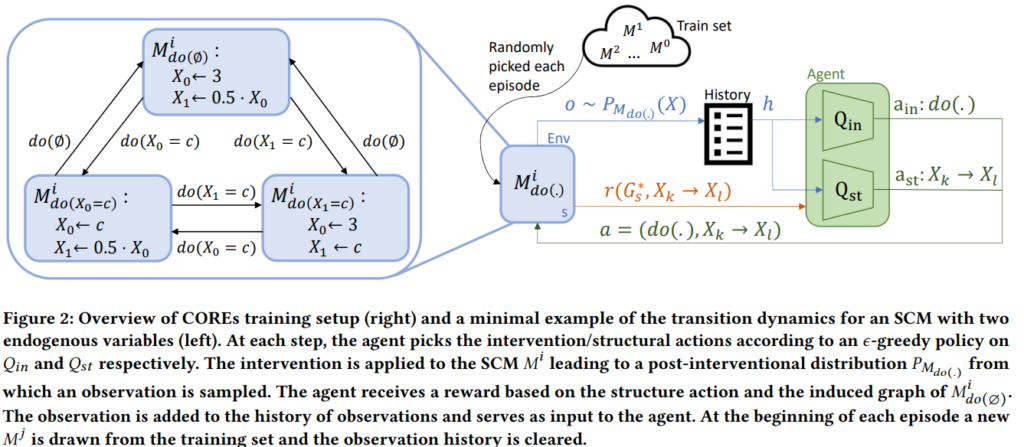
By training it on a broad spectrum of graphs, the learned policy can be applied even if the current graph was not encountered before. We empirically show, that there is a performance benefit when learning structure estimation in conjunction with the intervention schedule.
Compared to the SOTA, our approach scales to more nodes, trains faster, and provides more accurate graph estimates. While this is promising for causal discovery in real-world scenarios there are limitations of our approach w.r.t. to the applicability to complex causal relations.