I presented our work “A Meta-Reinforcement Learning Algorithm for Causal Discovery” at the workshop for Causal Representation Learning @UAI22.
I am happy that I could present our paper “A Meta-Reinforcement Learning Algorithm for Causal Discovery” as a poster at the Causal Representation Learning workshop at UAI22. I’m especially thrilled since this is my very first scientific piece of work. Here is an overview of the contents:
Knowing the causal structure of an environment is crucial for going beyond correlation-based predictions and allows us e.g. infer the effects of actions and draw conclusions from counterfactual worlds. However, estimating causal structures from data is inherently challenging.
In our approach, we train an agent to estimate the environment’s causal structure by experimenting on the environment. Even when applied to environments with unknown structures, that our agent has never seen before, our approach generates good estimates.
This holds true, even if we prohibit the agent to perform experiments. Nevertheless, in an ablation study, we show that experimenting greatly improves our agent’s performance. Overall, this is the first step toward an agent that determines causal structures for any environment.
I want to thank my co-authors and supervisors Erman Acar and Vincent François-Lavet for the great guidance. I also want to thank the participants of the workshop who engaged with me in great discussions. I am very excited to continue and exted this work in the next few months.
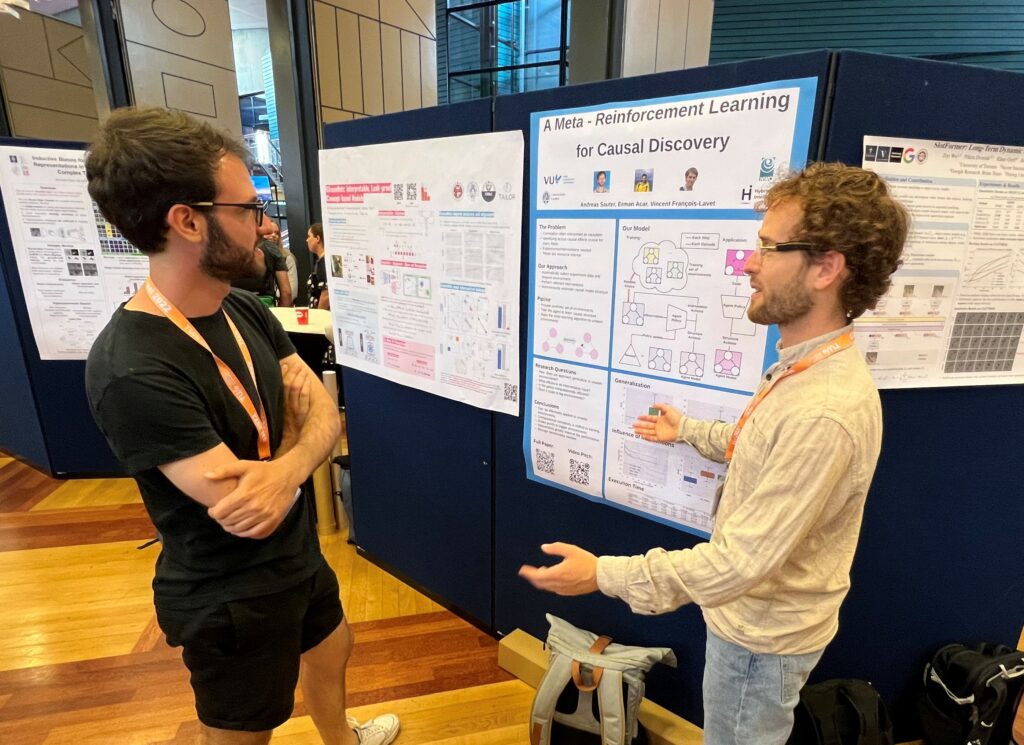